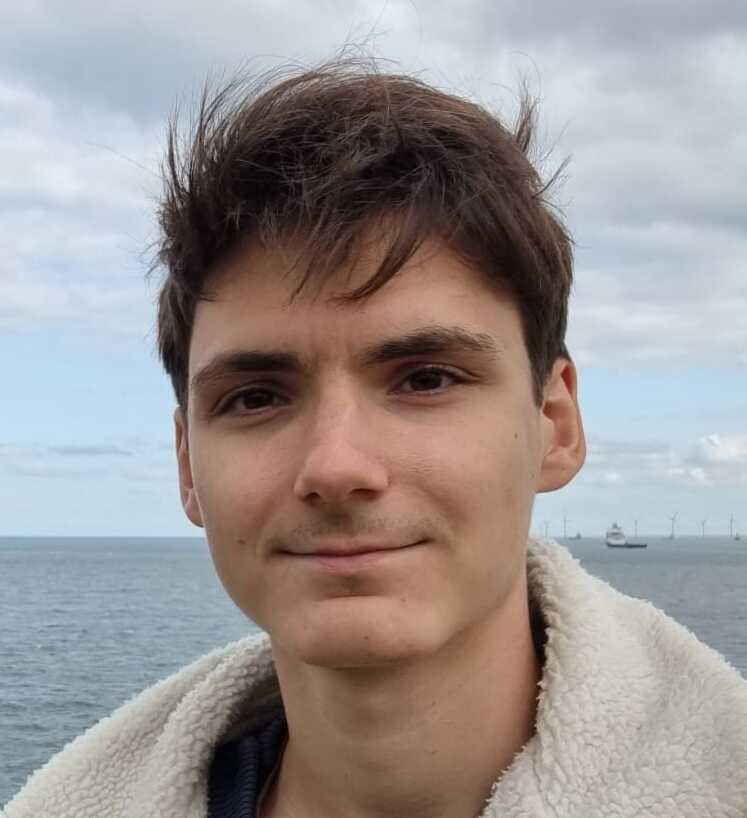
Hi! I am a second year PhD student at Mila and Université de Montréal, DIRO under the supervision of Gauthier Gidel and Courtney Paquette. Before my PhD, I studied Mathematics and Physics at Ecole Normale Supérieure, Paris and graduated from the Master Mathématiques de l'aléatoire, Orsay .
Research
My main interest is high-dimensional optimization, with a focus on stochastic gradient descent on simplified problems. The long term goal is to better understand the training dynamics of neural networks. My previous works mainly focused on loss landscape analysis, lottery ticket hypothesis, linear mode connectivity as well as generative models and alignement on human preferences.
Papers
Self-Consuming Generative Models with Curated Data Provably Optimize Human Preferences
NeurIPS 2024 (spotlight).
A theoretical study of generative models trained on synthetic data which is curated through human preferences.
Proving Linear Mode Connectivity of Neural Networks via Optimal Transport
AISTATS 2024.
We show that wide two-layer networks trained with SGD or multi-layer networks with iid weights can be linked in parameter space by low loss paths modulo a permutation of the neurons.
A General Framework for Proving the Equivariant Strong Lottery Ticket Hypothesis
ICLR 2023.
We study the existence of sparse subnetworks within overparametrized equivariant networks, that can approximate any smaller equivariant network.